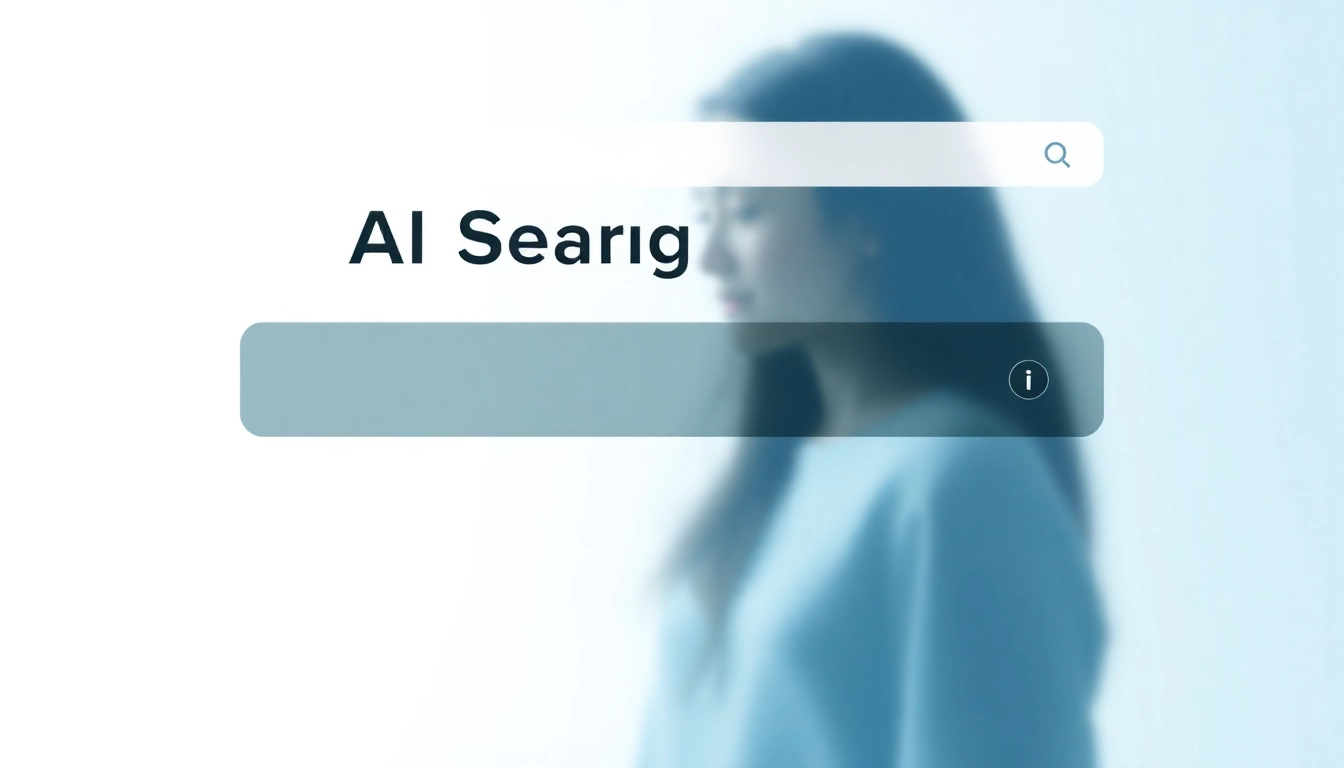
Understanding AI Search Technology
In today’s digital landscape, the way users interact with information is rapidly evolving, thanks in large part to advancements in AI search technology. These sophisticated search engines leverage artificial intelligence to understand user intent, context, and semantics, allowing for a more intuitive interaction compared to traditional search methods. This article explores the intricacies of AI search technology, its core components, benefits, integration strategies for businesses, and its future in the digital realm.
What is AI Search?
AI search refers to the use of artificial intelligence techniques and algorithms to improve search engine functionality. Unlike conventional search engines that rely primarily on keyword matching, AI search engines utilize machine learning, natural language processing (NLP), and advanced algorithms to analyze user queries in a more sophisticated manner. This approach allows them to provide more relevant, context-aware results. Typically, AI search systems can understand the nuances of natural language, providing users with information that is directly related to their queries rather than just retrieving results based on matching keywords.
How AI Search Differs from Traditional Search
Traditional search engines utilize algorithms that are heavily reliant on keywords and backlinks. Users must frame their queries in a manner that aligns with the search engine’s index, often leading to frustrations due to the lack of contextual understanding. In contrast, AI search engines comprehend the intent behind user searches. For example, if a user queries “best places to eat in New York,” AI search can analyze not only the words but also the underlying context, previous searches, and even trends in user preferences. This leads to results that are not only relevant but personalized, enhancing user experience.
The Evolution of AI Search Engines
The evolution of AI search engines has been marked by significant milestones over the last decade. In the early stages, search engines relied on basic algorithms and rudimentary scanning of web content. The introduction of machine learning transformed this landscape by enabling search engines to learn from user interactions. More recently, developments in natural language processing and big data analytics have further enhanced AI search, allowing for complex queries to be processed with high accuracy. Today, leading companies like Google and Microsoft are investing heavily in AI search technologies to provide users with more engaging and meaningful searches.
Core Components of AI Search Engines
Natural Language Processing in AI Search
Natural Language Processing (NLP) is a cornerstone of AI search technology. It enables machines to understand and interpret human language in a way that is valuable for search applications. NLP techniques analyze text to comprehend the context, intent, and sentiment, allowing AI search engines to deliver results that resonate with user queries. Approaches like tokenization, named entity recognition, and syntactic parsing are utilized to dissect user input and provide accurate responses. For instance, when users ask a question in natural language, NLP helps the AI search engine decode that question into a structured format it can work with effectively.
Machine Learning and Its Role
Machine learning plays a crucial role in ensuring continuous improvement in AI search capabilities. By training algorithms on vast datasets, AI systems learn to recognize patterns and nuances in user behavior and content relevance over time. This adaptability means that the more an AI search engine is used, the better it becomes at predicting user needs. Machine learning models can employ supervised learning, unsupervised learning, and reinforcement learning techniques to enhance search accuracy and relevance. As users engage more with AI search engines, they refine their learning algorithms to serve personalized results more effectively.
Data Structures for Efficient Retrieval
Efficient data retrieval in AI search engines relies on optimized data structures that can handle vast amounts of information swiftly. Commonly used structures include inverted indexes, which map content words to their locations in documents, allowing for quick lookups. In addition, graph databases can represent relationships and interconnections between different pieces of data, enhancing the AI search engine’s capability to return contextual results. By combining various data structures, AI search engines can provide comprehensive responses in a fraction of a second—delivering both speed and accuracy.
Benefits of Using AI Search Solutions
Improved User Experience
One of the most significant benefits of implementing AI search solutions is the improvement in user experience. Traditional search engines can often lead users to irrelevant results, causing frustration. With AI search, users receive personalized, context-aware results tailored to their unique preferences and search history. This enhanced experience not only increases user satisfaction but also encourages engagement, as users are more likely to return to platforms that consistently provide relevant information.
Instantaneous Results and Accuracy
AI search solutions can return results almost instantaneously, thanks to their ability to process information quickly and efficiently. As technology advances, the accuracy of the results continues to improve as well. With machine learning algorithms analyzing extensive datasets, AI search engines can refine their result generation approaches to produce highly accurate outcomes. This accuracy is crucial for businesses needing dependable information to make informed decisions based on user queries.
Personalization and Relevance
As AI search engines derive insights from user behavior, they can curate a personalized search experience. By analyzing previous interactions, these systems tailor results that align with user preferences, boosting relevance. For example, if a user frequently searches for vegetarian recipes, they will receive more targeted suggestions for similar content. This emphasis on personalization can significantly enhance user retention and loyalty, driving more conversions for businesses leveraging AI search technology.
Integrating AI Search into Your Business
Identifying Use Cases
Before implementing AI search technology, businesses must identify specific use cases that can benefit from such solutions. This could involve automating customer support, enhancing product discovery on e-commerce websites, or providing critical data insights for teams. By pinpointing these use cases, businesses can tailor their AI search solutions to meet specific goals, ultimately maximizing ROI. Common scenarios include improving website search functions, optimizing internal knowledge bases, or delivering personalized content recommendations.
Implementation Best Practices
Implementing AI search solutions necessitates a strategic approach. Firstly, ensure a clear understanding of your organization’s objectives and how AI search can address them. Collaboration between IT, content creators, and business stakeholders is crucial to gather the right datasets and feedback throughout the development process. It’s also important to invest in quality training data, as the effectiveness of AI search hinges on the datasets used during machine learning. Furthermore, continually test and iterate on your AI search capabilities to refine them according to user feedback and evolving business needs.
Measuring Performance and ROI
After integrating AI search into your business, monitoring its performance and ROI is essential. Metrics such as user engagement rates, click-through rates, conversion rates, and overall search satisfaction can provide insights into the effectiveness of the AI search solution. Regular analysis of these metrics allows businesses to adapt their strategies accordingly and refine the AI search technology to ensure it remains aligned with user and organizational needs. This feedback loop plays a significant role in the continuous improvement of AI search capabilities.
The Future of AI Search Engines
Emerging Trends and Predictions
The future of AI search engines is poised for exciting developments as technology continues to advance. Emerging trends include the incorporation of multimodal search capabilities that allow users to input queries through various formats, such as images, text, or voice. Additionally, voice search will become increasingly prevalent, necessitating further advancements in natural language understanding and processing. Businesses may also leverage augmented reality (AR) and virtual reality (VR) technology to create immersive search experiences, fundamentally transforming the way users interact with technology.
Challenges Facing AI Search Innovations
Despite the promising future, AI search solutions come with a set of challenges. Data privacy and security concerns are at the forefront, as users demand enhanced transparency on how their data is used. Additionally, finding the balance between automation and human oversight remains a significant challenge. AI search algorithms must emphasize ethical considerations and ensure they do not propagate biases that may inadvertently emerge during training. Technical hurdles regarding infrastructure compatibility and scalability also pose challenges as businesses seek to adopt advanced AI search technologies.
Preparing for Future Developments
To stay competitive, organizations must prepare for ongoing changes in AI search technology. This includes investing in continuous training for employees on AI functionalities and best practices. Additionally, fostering a culture of innovation within the organization can encourage exploration of AI applications that evolve with user demands. Finally, actively engaging with advancements in AI research will ensure that organizations remain on the cutting edge of search technology.